Conversations #84: Reimagining Leadership in Machine Learning
Written by WWC Team
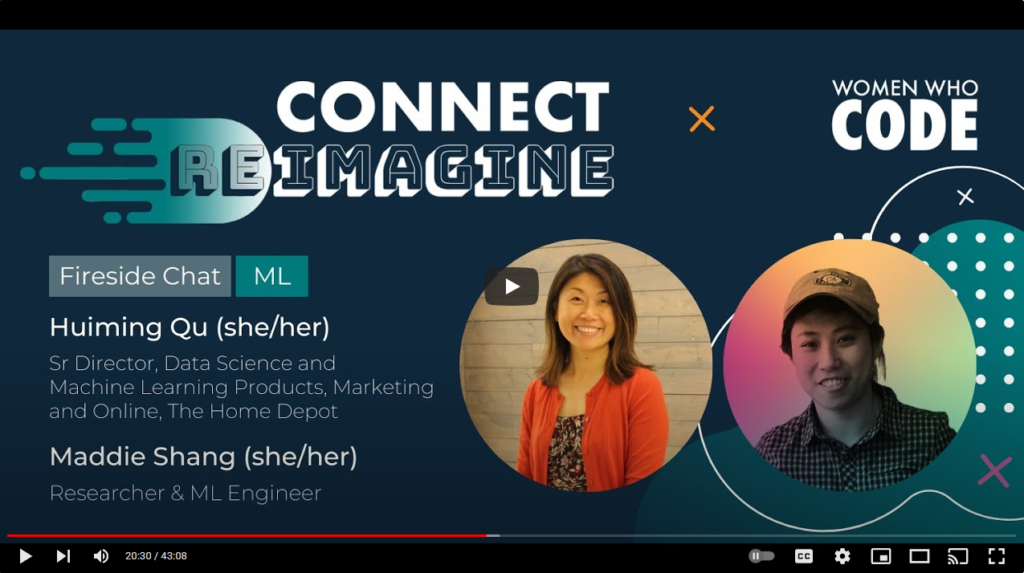
YouTube – iTunes – Spotify – Google – Podcast Page
Madeleine Shang, Co-Founder at Backflip Inc. and Data Science and ML Leadership Fellow with Women Who Code, sits down with Huiming Qu, Vice President, Data Science, E-Commerce, and Marketing at The Home Depot. They discuss Huiming’s journey to machine learning, advice for others on this path, and her advice for imposter syndrome.
How has working remotely in the current world changed how you and your team work? What are some of the challenges, solutions and bonuses of working remotely?
There are a lot of adjustments that have happened. One of the good things that came out of it is making the distance equal. We are distributed companies. We’re a distributed team. We have satellite teams in Austin, Dallas, and the Bay Area. At different places, people work remotely and sometimes feel they’re not as connected as those of us in the conference room. This actually brings everyone together the same. Another positive is the work-life balance. I thoroughly enjoyed the time saved not needing to commute.
What major decisions and turning points in your career led you to become a machine learning leader?
My soul-searching journey or career path is a series of little accidents. My academic background is in databases. Data management is working with data, real time data. I took a class of data mining from Professor Chris Felosis. He’s a great guy, very inspirational and just a very cheerful person. He got me interested in data mining and fascinated by some of the insights, patterns and problems that we can solve using machine learning and data mining. I entered into my first job in IBM Research and didn’t do anything I learned in my PhD thesis. It’s all about actually solving problems. I joined the service department solving problems using machine learning, data mining skills, and optimization. It took a few to realize that I wanted to solve the problem end-to-end.
Do you have any advice or resources to point to for people who are considering becoming an AI leader?
Be less anxious. Embrace all the experiences that you have. Embrace whatever you’re given, whether extreme happiness or sadness. It’s all going to be adding wisdom to yourself.
Are there opportunities for entry if there’s no formal machine learning background? Do you recommend going through a traditional pass, getting a master’s or doctorate?
We all have to start somewhere. The starting point could be now, it could be any point of your experience. It really doesn’t need to be traditional formal training. You must appreciate the experience with technical knowledge. I think it’s easier in an academic setting. You may come from a psychology background or social study, giving you a perspective to connect with people or solve specific problems. Leverage the strength of your background and then from there, there are many tools and materials. We need to train ourselves with all kinds of data that will help really mimic the real world.
What are your key success criteria for being a leader in machine learning for a big company?
The success criteria for the team is not so much different from the success criteria for every team member. If you really add that up, the success for the team should be every team member’s success and then it’s vice versa. I don’t think there’s going to be an equation. Everyone is successful when the team is. So striving for everyone’s success is essentially how we cascade it.
When did you last notice imposter syndrome in yourself as a leader or in one of your team members? And how did you go about resolving and dealing with these things that happened?
After I record a podcast, I don’t want to listen to my voice. I’m a perfectionist and lack of confidence contributes to imposter syndrome. For those of you feeling uncomfortable, I tell myself to fake it until I make it. Whatever the problem that you are focusing on nobody knows better than you. Sometimes even when you don’t believe it, you can still fake it and people will believe it. They only see from the outside until you get the time to really grow into that image that you want it to become. Whatever you feel you’re not good enough, that’s an internal drive for you to get better and you may have a really high bar. We all do, and many of our women have that high bar to get there. It’s a gift.
Why are diversity and inclusion important in terms of ML AI Data science?
I have seen great intention within Home Depot and other places I have worked. We have to be intentional. This will be the most important thing if you look at it from a machine-learning perspective. We all know the importance of stratification. You don’t want to build a data set for the famous machine learning example that image recognition is best for a white male. The dataset is actually biased. Create good ideas and have those benefits of the intelligence from all people to contribute to your project, your organization, the health of long-term projects and the company. This is very important for the success of the company. From an individual and corporate level, we can all do better to improve and move the needles towards the right direction.